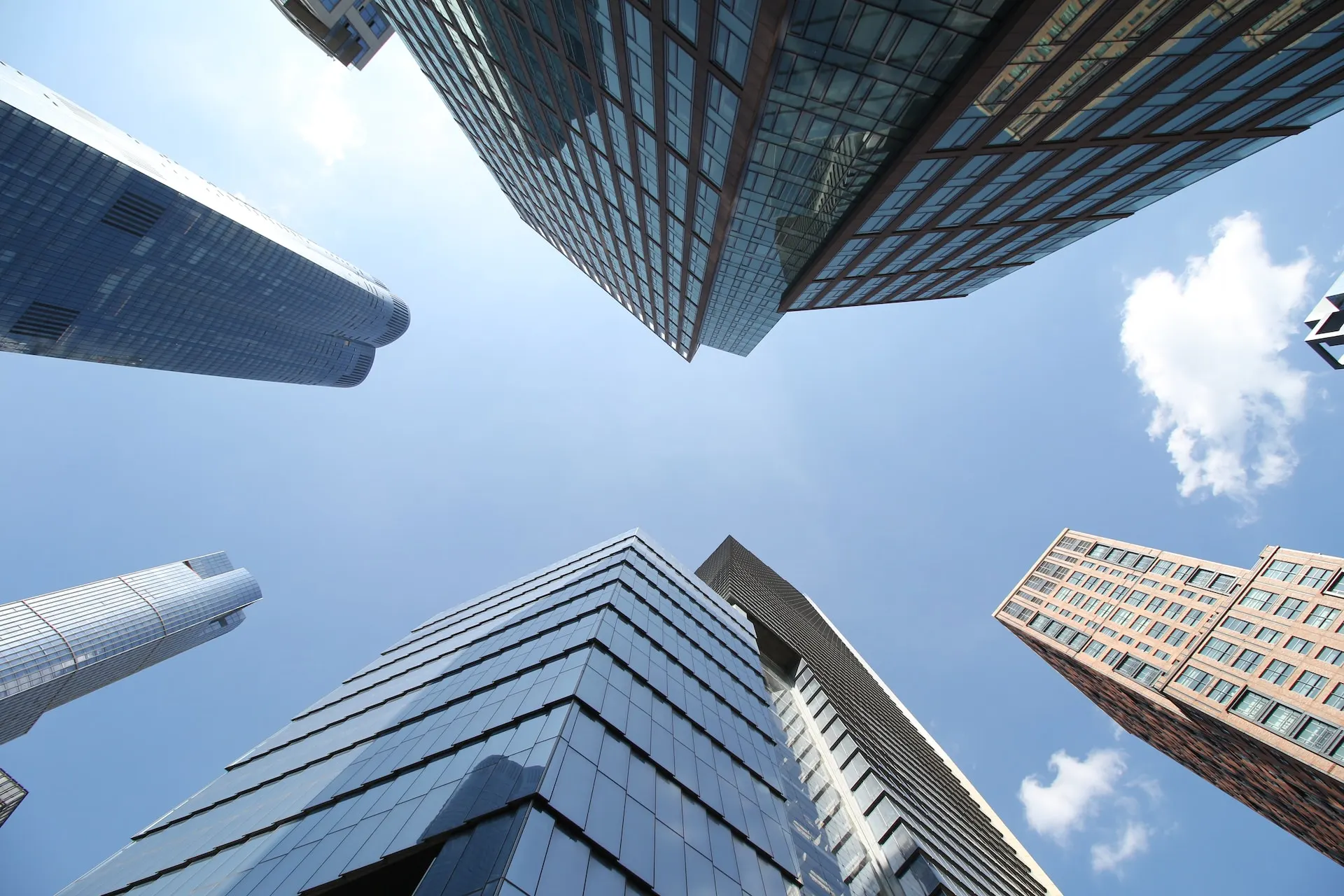
The investment world is buzzing with talk of generative AI, but the reality on the ground is more complex than headlines suggest. When ChatGPT debuted, it didn't just make waves—it triggered a tidal shift in how investment firms approach technology risk. Suddenly, "Gen AI risk" became a staple page in IC memos, reflecting a new era of technological uncertainty.
Private equity investors now find themselves in a peculiar position, with AI acting both as a potential goldmine or a minefield for new deals and portfolio companies. Investment firms scrambled to form "AI committees" and acquire new tools to avoid falling behind in what appeared to be a paradigm shift in the industry.
In this inaugural "State of AI in Investing" report, we'll focus on the current state of AI adoption in large cap private equity firms (those with $50B+ AUM or writing $1B+ equity checks) and summarize our view on the broader “AI for alternatives” category. Our insights are based on in-depth discussions with over 120 investment professionals, including 40+ individuals from 15 large cap PE firms.
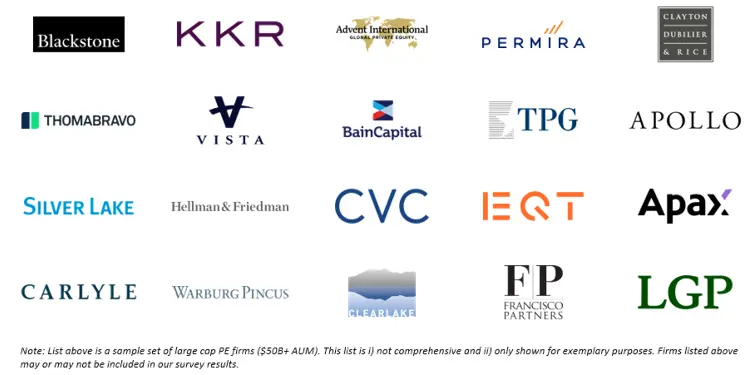
Summary across large cap PE firms

Large Cap PE ($50B+ AUM) Benchmarking
In-house Development
Approximately 20% of large cap PE firms have developed proprietary Gen AI and portfolio monitoring tools. A small minority of firms (c.13%) have deal modelling software, and nearly half (40%) have in-house data science teams.
Public vs. Private Firms
Publicly traded large cap firms typically possess more advanced in-house data science capabilities (60% with in-house teams) vs. privately held ones (30% with in-house teams), often with dedicated teams of 3-8 people.
AI Governance
The vast majority (80%+) of firms across both public and private sectors have established AI committees.
Gen AI Adoption
The majority of large cap PE firms have experimented with at least one Gen AI solution. However, the general sentiment suggests that these tools are not meeting expectations.
Select Quotes
I’ve tested out every single AI-powered diligence provider out there, from [Provider 1] to [Provider2] to [Provider 3]. At best, these tools are as useful as a sophomore intern putting together a first cut for you. Every single time, I’ve ended up recutting the materials myself – Large Cap PE Senior Associate
We’re a paying customer of [Provider 1]. No one really uses the tool, but our IT team keeps telling us that we are getting a great deal, so I guess we are still using it. – Large Cap PE Vice President
Our in-house [sourcing /gen AI] tool is not very helpful. It’s a less sophisticated [version of] Deal Cloud that is not very user friendly. – Large Cap PE Associate
We just onboarded [Provider 1] last week. It’s super slow and I might as well just look for the data myself. I don’t even think summarizing a CIM is useful – because that’s my job anyways. My current reaction is that I don’t know if it’s a workflow tool for me, because I need to do the work myself. – Large Cap PE Associate
I love [our deal modelling tool]. It instantly builds a model and the important outputs for any public company based off consensus estimates. Saves me hours from having to build it all myself. – Large Cap PE Associate
We’ve been inundated by LP question on Gen AI and how we are addressing this risk – that’s why we’ve onboarded [Provider 1], so that we are always up to speed on what’s going on in the market. – Large Cap PE Partner
Large Cap PE Survey Key Findings
Most firms pay out of defensiveness than true utility today
- There is significant pressure from LPs to GPs to understand Gen AI and risks, and the intention of most firm spend is to not be “behind the curve”
- Despite minimal usage of these tools, firms are willing to allocate dollars to i) have a pulse on the latest developments in Gen AI land and ii) inform their internal strategy of tooling to build
- In general, large cap firms tend to be more reactive rather than proactive. Firms tend to think more about what competitors are using rather than doing the tedious work for market mapping and truly understanding the functionality of the available tools
There are 4 key tools that large cap PE firms build in-house
[1] Data Science
- Out of internal teams, these are most frequently cited as the greatest value add for both deal teams to cut large swathes of data as well as portfolio operations teams
- These teams are much more prevalent in crossover / hedge funds, which we will break down in a subsequent write-up
[2] Gen AI
- There is a small handful of investment firms who have built Gen AI tools in-house (oftentimes they are also paying customers of other solutions)
- These tools tend to be relatively similar clones of ChatGPT or a chatbot in which you can securely chat with your enterprise data
- While a great marketing endeavor to LPs, typically there is low usage from the investment professional side
[3] Deal Modeling
- A small handful of large cap PE firms have built their in-house deal modeling tools. These tools have high NPS and are used frequently for early stages in deals
- While these tools are less customizable, and more oriented towards forming an 80/20 view, the feedback we’ve received from investment professionals is that is quite useful for low probability deals in which investment professionals will need to “do the work”, but not necessarily go to investment committee / hire external advisors
[4] Portfolio Tracking
- These tools generally have high NPS, as it becomes the single source of truth for every portfolio company at the PE firm, and is the medium by which investment professionals / IR teams pull data for reporting purposes
- Firms typically spend between$2-5M a year to build, and then $500K+ / year to maintain
There is no external AI tooling that is ingrained in the investor workflow today
- While there are Gen AI players that have penetrated most firms from a POC / GTM perspective, there has generally been high churn, low usage, and low NPS / mixed reviews based off our 40+ user interviews
- While many companies may have shiny demo’s, the true use case for investment professionals today is still quite limited today given the high degree of accuracy needed and dire consequences of a mistake
Our Thoughts
The "AI for alternative investments" category is in its infancy. We estimate current spending on external Gen AI solutions is less than $20 million of spend in large cap PE and under $50 million across all private equity today. Most of the developments in the category have been driven by improvements in the underlying foundational models, rather than true end-to-end automation and any useful application layer being built.
Outside of finance, other use cases such as legal are much more apparent, and this is a result of the functionalities of large language models (LLMs) today that power all these AI tools. Foundational models are excellent at text summarization, knowledge retrieval, sentiment analysis, and basic conversation. What they are not capable of, however, is complex reasoning, logic, math, and nuanced decision making – all of which are the crux of the job of the investment professional.
To investors, it seemed that ChatGPT came out of nowhere, but in reality, it was the culmination of over a decade’s worth of work from some of the most brilliant minds in AI/ML. It is natural to overestimate what can be done in a few months, and underestimate what can be done in a few years. There is clearly a meaningful gap between expectations of Gen AI and the reality of what these tools can do. While Gen AI might be able to answer one-off questions on public filings or internal documents, today, it cannot automate highly fidelity tasks such as private equity style diligence, or even sourcing new deals.
Investment professionals are naturally taught to be logical, linear thinkers. To an investor, the gap between summarizing a CIM to generating a first cut of buyout private equity style diligence materials may not seem significant, but the intricacies of making that a practical reality are much more complex.
At Prosights, we recognize the current limitations of generative AI, which is why we prioritize perfecting low-fidelity, monotonous tasks like search, chat, and data tracking to ensure reliability and efficiency for investment professionals. This approach allows us to build a robust foundation and be well-prepared to handle more domain-specific, agentic tasks as the capabilities of foundational models improve. We’re collaborating directly with the leading foundational model providers, including OpenAI and Anthropic, and are eager to apply the latest models as they become available.
In subsequent write-ups, we’ll discuss topics such as the landscape of AI software for investors, Gen AI risks for portfolio companies, and AI adoption / strategies across broader PE, crossover / hedge funds, venture capital, and secondaries. Subscribe here to our newsletter to stay up to date.
For personalized guidance on understanding how you stack up versus other PE firms Gen AI adoption please reach out to our expert team at founders@prosights.co.
Exhibits
[1] Survey results for large cap PE Firms #1-5

[2] Survey results for large cap PE Firms #6-10

[3] Survey results for large cap PE Firms #11-15
.png)